Generative AI for Risk Management: The New Way to Compute VAR Using Model-Free Frameworks
1/16/20244 min read
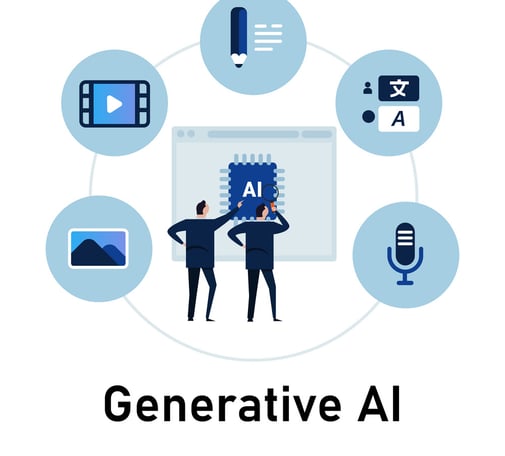
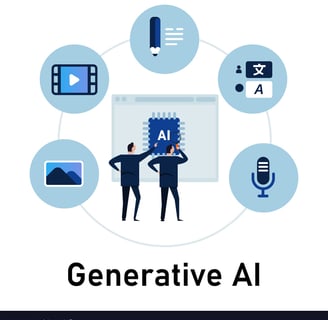
Risk management plays a crucial role in the financial industry, helping organizations identify and mitigate potential threats to their financial stability. One of the key measures used in risk management is Value at Risk (VAR), which quantifies the potential loss that an investment portfolio may incur under adverse market conditions. Traditionally, VAR has been computed using statistical models that rely on assumptions about the underlying data distribution. However, with the advent of generative Artificial Intelligence (AI), a new approach to computing VAR has emerged.
Generative AI refers to the use of AI algorithms to generate new data that resembles a given dataset. This approach is particularly useful in risk management, as it allows organizations to simulate various market scenarios and assess the potential impact on their portfolios. By generating synthetic data that captures the risk characteristics of the underlying assets, generative AI networks like GANs enables risk managers to compute VAR without relying on specific stochastic models. Deep Learning Networks are model-free frameworks are provide a flexible and adaptive approach to risk management.
Unlike traditional models, which make assumptions about the data distribution, model-free frameworks learn directly from the data, allowing for more accurate and dynamic risk assessments. By combining generative AI with model-free frameworks, organizations can harness the power of machine learning to compute VAR in a more robust and efficient manner. One of the key advantages of using generative AI for risk management is the ability to capture complex dependencies and non-linear relationships in the data.
Traditional statistical models like normal distributions often struggle to capture the tail risks of equities, leading to inaccurate risk assessments. Generative AI, on the other hand, can learn from the underlying data distribution and generate synthetic data that accurately reflects the risk characteristics of the portfolio. In our research, we harness the advanced capabilities of Generative Adversarial Networks (GANs) to analyze and replicate the complex patterns of the S&P 500 index over a period spanning from 2000 to 2021. GANs, a breakthrough in the field of deep learning, consist of two distinct but interconnected neural network models: the generator and the discriminator.
The generator's primary function is to create synthetic data that closely resembles the actual S&P 500 index data. It does this by learning the underlying distribution of the real data. The generator starts with a random noise input and progressively refines its output through the training process, attempting to produce data points that are indistinguishable from the real stock market data.
Conversely, the discriminator acts as a critical evaluator. Its role is to distinguish between the real data points from the S&P 500 index and the synthetic data generated by the generator. During training, the discriminator is continually challenged to improve its accuracy in identifying authentic data versus the increasingly convincing synthetic data produced by the generator.
This dynamic competition forms the core of a GAN’s training process. The generator and discriminator are in a constant battle, with the generator striving to produce more realistic data and the discriminator becoming more adept at spotting fakes. This adversarial process is critical as it drives both networks towards greater levels of performance. The generator learns to produce more accurate representations of the stock market data, while the discriminator becomes more efficient in distinguishing real data from forgeries.
In constructing our GAN model for the S&P 500 index, we faced numerous challenges. A pivotal aspect was the architecture selection for both the generator and discriminator. This involved determining the number of layers, the type of layers (such as convolutional layers for feature extraction), and the connections between these layers. Another crucial aspect was the selection of optimization algorithms and tuning of hyperparameters, which are essential for guiding the learning process and ensuring convergence of the model.
Our model is built on a foundation of over 200,000 parameters, which underlines its complexity and capability to handle intricate patterns in financial data. The use of convolutional networks is particularly noteworthy. Unlike traditional neural networks, convolutional networks are adept at identifying and leveraging spatial hierarchies in data, making them exceptionally suited for tasks where data points have a high degree of interrelation, as is often the case in financial time series.
Through this advanced GAN framework, we aim to provide a nuanced understanding of the S&P 500 index’s behavior over the past two decades, uncovering patterns that might not be immediately evident through conventional analysis methods. This could offer valuable insights for financial forecasting, risk management, and investment strategy formulation.
In the chart presented, we can see the comparison between the original distribution and the one generated by the GAN network. The similarities are quite remarkable. The skewness of the generated distribution stands at -0.33, closely approaching the actual skewness of -0.22. While the true kurtosis is at 10, the GAN-produced kurtosis is not far behind at 5.2. These results are notably impressive, showcasing the effectiveness of the GAN's deep architecture and its use of convolutional networks to intricately mimic the SPY500's distribution.
The successful replication of the return distribution holds significant potential for various financial applications, such as derivative pricing and risk metric computation. Particularly, it enables the generation of precise Value at Risk (VaR) measurements by simulating market behaviors that are nearly identical to real-world scenarios. This is a notable advancement over traditional VaR models that rely on Normal distributions, as GANs adeptly account for the heavy tails in the distribution, integrating them seamlessly into their calculations.
In conclusion, generative AI and model-free frameworks offer a new way to compute VAR in risk management. By harnessing the power of machine learning and generating synthetic data, organizations can obtain more accurate and dynamic risk assessments. This enables risk managers to make informed decisions and develop effective risk mitigation strategies. However, it is important to approach the implementation of generative AI with caution and ensure that the generated data accurately reflects the underlying risk characteristics. With the right expertise and careful consideration, generative AI can revolutionize risk management and enhance the financial stability of organizations.
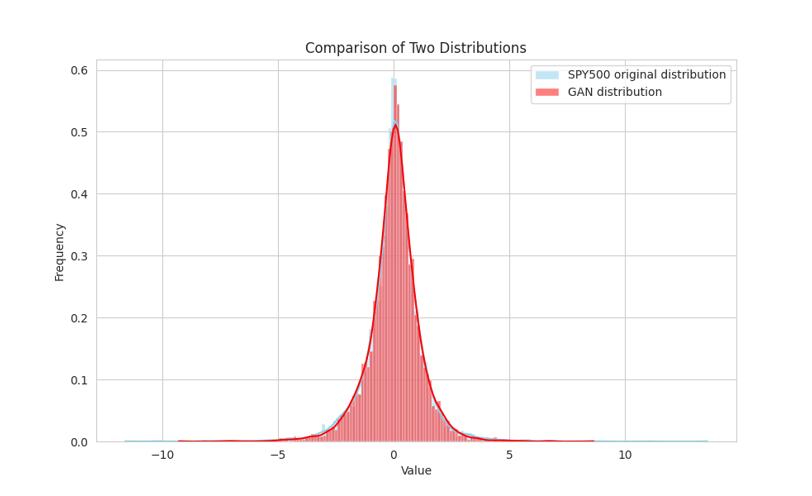
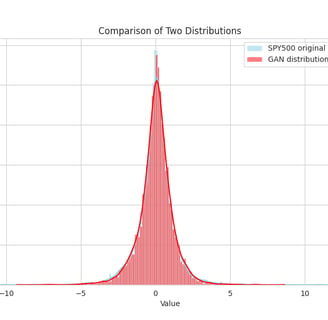